Do you know what is the application with the fastest-growing user base ever?
Hint: it is not TikTok. This social media phenomenon was relegated to second place earlier this year by ChatGPT. ChatGPT achieved the milestone of 100 million monthly active users in a mere two months, while TikTok needed then record-breaking nine months. Instagram needed two and a half years for the same.
Generative AI surely had its TikTok moment as colleague Dan Miller of Opus Research formulated it in a CRMKonvo.
This is a testament to two things. First, in contrast to some earlier hype topics that never reached a broad usership, generative AI actually seems to help solve real problems. In contrast, Metaverse, NFT, and cryptocurrencies seem to be rather solutions in search of a problem.
Second, it is easy to use; as easy as Google search.
The Ugly
Now, generative AI tools require massive amounts of compute resources, during training as well as during runtime. While research on how to reduce this is going on, there are estimates that a single training run of GPT3 costs more than 4.5 million USD. Estimates vary, but one does need multiple runs, each of which can take considerable time. In brief, one can say that it costs a lot and that it is depending on the model’s size. This means that only big companies can meaningfully provide these tools, or train an instance for a specific purpose.
It also means that these models cannot (yet) be retrained frequently. This has some repercussions when it comes to their application to volatile business environments.
Pricing is accordingly, usually based on tokens, which can be roughly equated to a short word or a syllable, in the case of text, or by image in the case of images. Although the token prices seem low (here the Aleph Alpha, Microsoft and OpenAI pricing pages), this can sum up quickly, as shown in this readable article by Steven Forth.
In addition, there are more and more regulatory bodies looking into privacy and IP issues that may arise from training and using LLMs. E.g, Italy has at least temporarily banned ChatGPT for GDPR reasons at the end of March 2023. Germany and other European countries, plus Canada, have launched at least a probe.
On top of that, IP protection is nothing less than a minefield. Can content that is generated by a generative AI be considered original and therefore be subject to IP protection? Or is a generative AI rather a derivative AI that at least partly uses original content, perhaps without permission, to create its results? This is one of the reasons for Adobe to emphasize on the ethically correct training of its upcoming Firefly product.
On top of this, it is also necessary to protect their own IP and internal data, sensitive or not. Using generative AI services might expose internal data to the public, depending on the use of the service. Samsung and Amazon, amongst other companies, recently found this out the hard way.
It is no good to feed corporate data or information into systems if you are not fully aware of what happens with this data or information. It is paramount to understand the terms and conditions of their use.
Given all this, the main consequence of these limitations and challenges is that businesses need to wisely choose where to apply generative AI and where to not do so. This does not mean that business challenges should not be addressed with the help of generative AI or at all, but that it is worthwhile looking at other technologies to solve the problem. Possible reasons for choosing another technology include cost or not making oneself vulnerable to litigation for privacy or IP protection reasons.
At the risk of sounding like a broken record: Not everything that can be solved using new technology needs to be solved using the new technology, tempting as it might be.
But then …
At the same time, the use of generative AI does provide benefits to employees as well as customers. There are a good number of scenarios that are already implemented and at least in beta use that show this. Employees can become more effective and efficient. They are rid of chores, which improves their experience. Customers can get issues resolved faster and in the way they communicate. This enhances their experience. I have written about this in my last column article How Business Leaders Can Leverage Generative AI in Customer and Employee Experience as well as in other posts on my blog, e.g, my article Beyond the Hype – how to use ChatGPT to create value.
So, what to do?
In brief: Generative AI is here to stay. Leverage the technology where useful while educating your employees about the risks that are associated with the use of freely available models.
The models will get even more powerful and with that potentially useful in more situations. On top of that, there is healthy competition already now, as you can see from the diagram below which shows a subset of the available models.
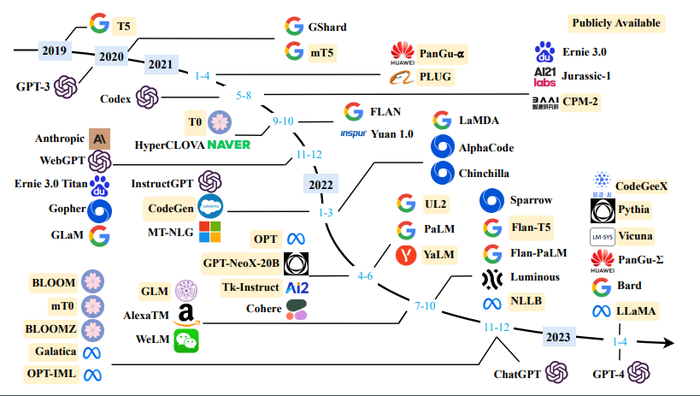
This competition, along with the fact that several of these models are open source, will lead to price reductions. Furthermore, there is a lot of research going on about how to make LLMs more efficient during training as well as during runtime. As an example, Graphcore and Aleph Alpha presented their findings in November 2022. Additionally, due to the increasing availability of pre-trained models, the necessity for full-fledged training gets greatly reduced. Instead, models only need to get fine-tuned to support the intended use cases. As fine-tuning only requires a task-specific data set which is far smaller than the data set necessary for general training (pre-training) of the model, this training is both faster and cheaper.
Along with an uptake of the technology, this means that the price tag for using generative AI can be expected to go down even in the near future.
Recommendations
First, establish clear guardrails and governance, augmented by training for the employees, for the use of generative AI technologies to help employees and protect the business. Employees need to be prepared to understand and assess the outputs of generative AI while policy should prevent them from using outputs that they do not understand and that do not come with appropriate references to source data or from using sensitive information on public models. While this is critically important, I will not dive deeper into it.
Second, identify use cases where a generative AI may be helpful to address a business challenge, be it a problem that needs to be addressed or a capability that shall get acquired.
From these use cases, select one or few that are reasonably important to solve and isolate. Depending on the skill sets that are available in the business, it might be better to initially focus on use cases that do not require training a model but can be solved by fine-tuning or just using an existing model.
For each of these use cases, create a business case, knowing that a generative AI may cause significant variable costs when in use. The business case must show clear KPIs that are to be improved, along with the monetary impact of this improvement. This is particularly important – albeit difficult – for CX use cases.
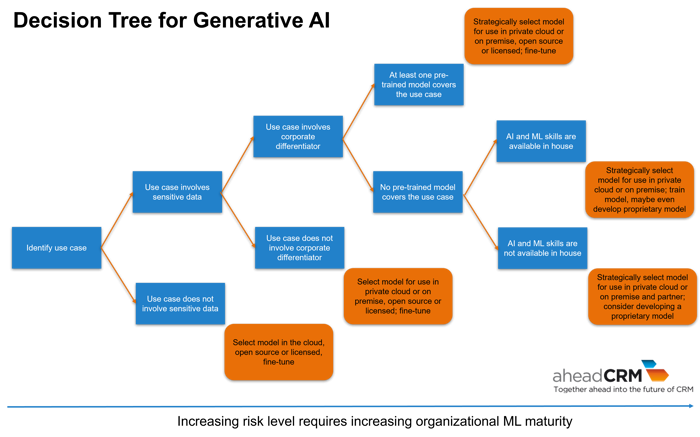
Although experimentation will remain important, it is equally important to identify models that fulfill as many of the identified use cases as possible. This helps avoid the necessity of using multiple models with all the overhead and cost this includes.
A part of the decision for one model or another is the sensitivity of the data that is to be used, whether or not the use case covers a capability that already does or shall become a corporate differentiator and what the AI and machine learning maturity of the organization is. Size does matter, too. It is unlikely that a small company has the capacity or the personnel with the necessary skills to develop its own proprietary model. Coupled with the appetite for risk the organization has, this also gives a sweet spot for decision-making.
Think big – act small. Find a use case that is reasonably relevant to start with while having in mind that you will want to fine-tune the selected model for additional purposes. Measure, learn, repeat, and rinse.